Background
‘Immortal time’ is when participants of a cohort study cannot experience the outcome during some period of follow-up time. When immortal time is misclassified or excluded during analysis, immortal time bias leads to a biased association. This usually happens when researchers assign participants to treated or exposed groups by using information that is observed after the participant enters the study (after time-zero). This is common in pharmacoepidemiological studies where there is a delay in classifying participants as ‘treated’ until they fill their first prescription some time after entering the study. Because these participants must have survived (be alive or event-free) the time between entering the cohort and filling their first prescription, they are considered ‘immortal’ and contribute ‘immortal time’ to the treated group by design. Bias is introduced when this period of ‘immortality’ is misclassified or excluded during analysis, resulting in a distortion of observed effects in favour of the treatment (or exposure) under study by conferring a spurious survival advantage to the treated (or exposed) group (Figure 1).
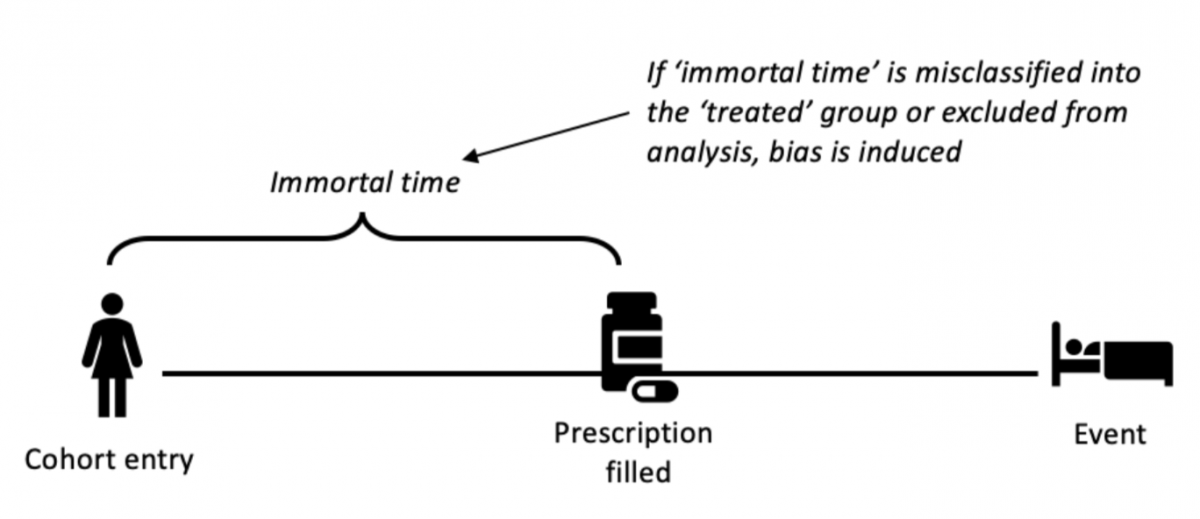
Figure 1. The structure of immortal time bias
Example
The earliest example of immortal time bias was recorded in 1972 by Gail in a study of heart transplantation.[1] Later, Suissa (2007) reviewed a selection of 20 examples in pharmacoepidemiology and more examples have surfaced since.[2]
To highlight one example, several observational studies reported that inhaled corticosteroids could effectively prevent readmission and mortality in patients previously hospitalised with COPD. In the original studies, immortal time bias was introduced because participants entered the cohort on the day they were discharged and were then assigned to the treated group if they filled a prescription for a corticosteroid within the first 90 days from discharge. By design, participants allocated to the treated group could not have died or been readmitted between the time of entering the cohort and the time of filling their first prescription. In effect, they contributed ‘immortal time’ to the treated group. The original studies misclassified this immortal person-time to the treated group (rather than the untreated group) and immortal time bias was induced (see Figure 2).[3]
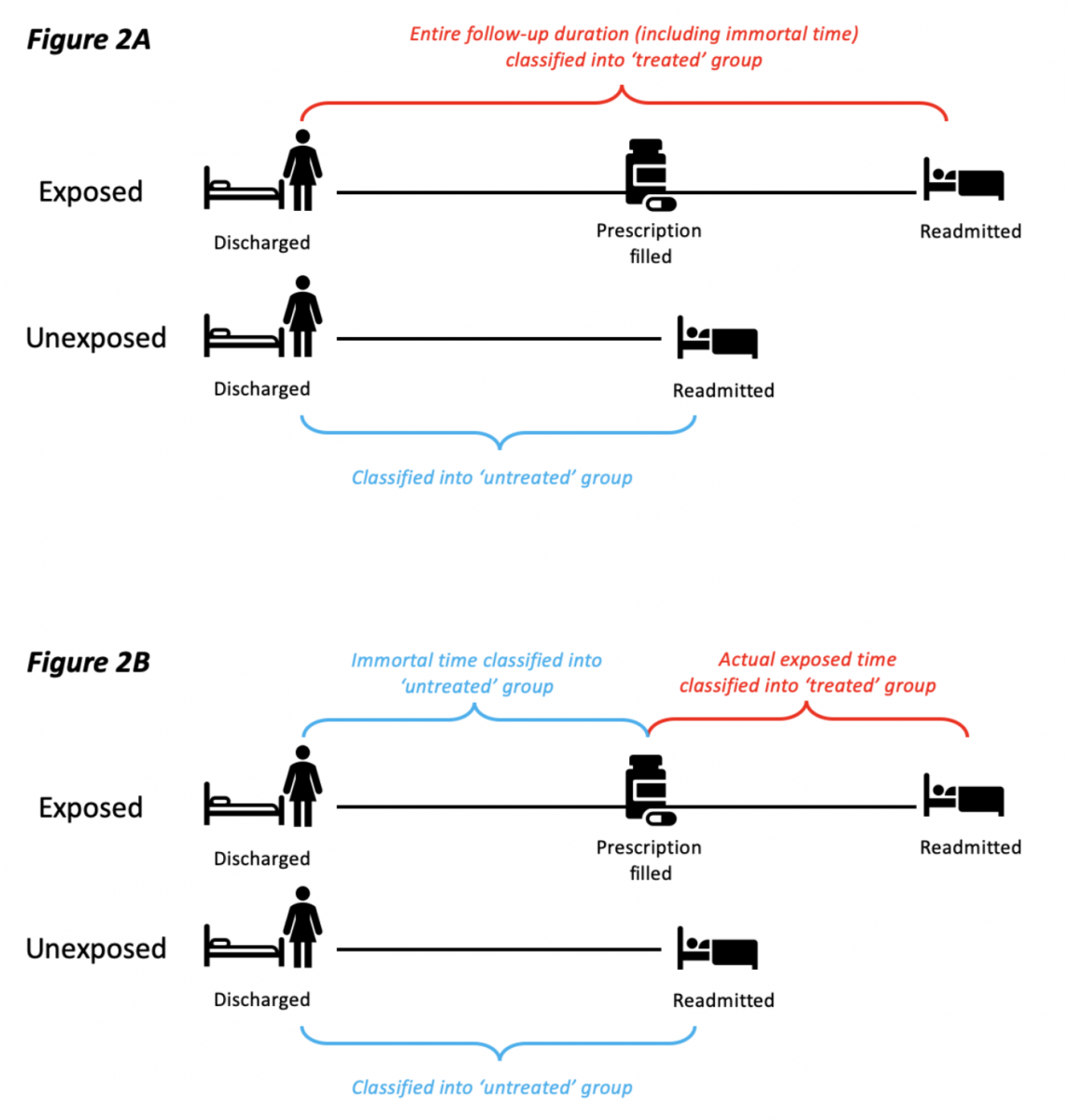
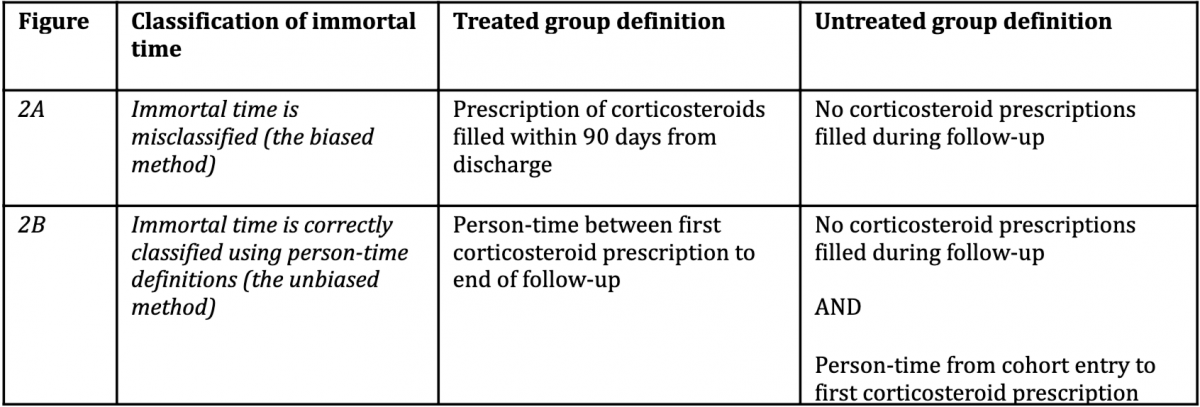
Impact
In the corticosteroid example, by misclassifying immortal person-time to the treated group, the original studies produced a rate ratio of 0.66 in favour of the treated group. Suissa’s new analysis that correctly reclassified immortal person-time to the untreated group produced a rate ratio of 0.79.[3]
Immortal time bias can also reverse conclusions. For example, a cohort study showed that statins could reduce the risk of diabetes progression (adjusted hazard ratio 0.74, 95% CI 0.58 to 0.95). But in this study, they misclassified immortal time and used a naive time-fixed analysis. A proper time-dependent analysis that correctly classified immortal person-time to the untreated group reversed the effect and revealed that the hazard ratio was 1.97 (1.53 to 2.52). This reanalysis also showed that the magnitude of immortal-time bias increases as the duration of immortal time increases.[4]
Preventive steps
The only way to prevent immortal time bias is by designing studies so that participants are assigned to exposure groups based on their data at time-zero, rather than their data after time-zero. In other words, assignment and time-zero must be aligned. If the study cannot be designed to avoid immortal time bias, then time-dependent analyses can be used to reduce its impact. These methods use person-time definitions to correctly classify participants who are in immortal-time into the unexposed group (rather than the exposed group). Although these analyses can be useful, they cannot fully eliminate immortal time bias and they also run the risk of inducing other types of biases.