Hopin Lee, Jeffrey K Aronson and David Nunan blog about how to tell when an association does and does not mean causation in health research
A statistical association between two variables merely implies that knowing the value of one variable provides information about the value of the other. It does not necessarily imply that one causes the other. Hence the mantra: “association is not causation.”
Suppose that we want to know if acute trauma to a joint (an exposure) causes chronic knee osteoarthritis (an outcome). Because it would be unethical to conduct an experiment whereby we deliberately inflict joint trauma to assess its effects on chronic knee osteoarthritis, we decide to tackle this question by using observational data from a hospital registry. Let’s say that, in this dataset, we detect an association between joint trauma and knee osteoarthritis. To claim that this association represents a causal effect, we need to first rule out two possible issues that lead to a non-causal association:
- Confounding
- Collider bias
Only if we can rule out these biases can we begin to think that association may imply causation.
Confounding
Confounding occurs when an exposure and an outcome share a common cause (the confounder; Figure 1). Failure to control for the confounder makes it appear that there is an association between the exposure and the outcome, whereas in fact both are caused by the confounder and are not related to each other at all (or as strongly).
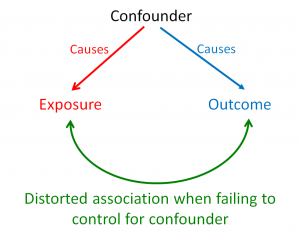
Figure 1. Causal diagram illustrating the structure of confounding.
In our example, it is plausible that joint trauma and knee osteoarthritis share a common cause – high impact sport (the confounder). That is, individuals involved in high impact sport may be more susceptible to both acute joint trauma and chronic knee osteoarthritis (through repeated use). In this case, if the confounder (high impact sport) is not controlled for in the data analysis, we will observe a distorted association between joint trauma and knee osteoarthritis (Figure 2).
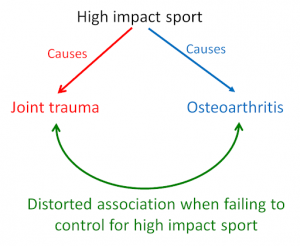
Figure 2. Causal diagram illustrating a distorted association between joint trauma and osteoarthritis through failure to control for the confounding factor, exposure to high impact sport.
Only by considering all possible confounders and adjusting for them (by study design or analysis), can we confidently claim that joint trauma causes knee osteoarthritis (that’s if we can also rule out other biases). However, it may not be possible to identify and measure all possible confounders in an observational dataset. So in practice, it becomes quite a challenge to make strong causal claims without controlling for unknown and unmeasured confounders. Methods for tackling these problems are beyond the scope of this blog.
Collider bias
Collider bias occurs when an exposure and outcome share a common effect (the collider). In this case, a distorted association between the exposure and the outcome is produced when we control for the collider, as illustrated in Figure 3.
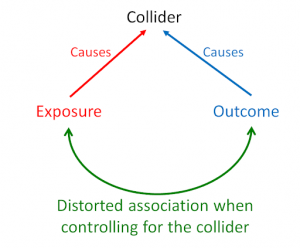
Figure 3. Causal diagram illustrating the structure of collider bias
It is plausible that both joint trauma and knee osteoarthritis lead to surgical intervention, such as knee arthroscopy (the collider). That is, individuals who suffer a traumatic joint injury or those with a diagnosis of knee osteoarthritis are likely to undergo knee arthroscopy. In this case, if the collider (knee arthroscopic surgery) is controlled for (by study design or analysis), we will observe a distorted association between joint trauma and knee osteoarthritis (Figure 4).
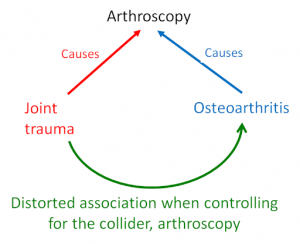
Figure 4. Causal diagram illustrating a distorted association between joint trauma and osteoarthritis by controlling for the collider, exposure to arthroscopic surgery
Collider bias could be induced if, for instance, researchers only gain access to data from those who have undergone surgical intervention (which would induce selection bias – a form of collider bias). Or if researchers have access to the entire dataset, but mistakenly decide to statistically control for surgical intervention during analysis. In effect, both mistakes will induce a biased association between joint trauma and knee osteoarthritis. When we study a group of individuals who received surgery (only as a result of joint trauma or knee osteoarthritis), knowing that a patient underwent surgery because of joint trauma will tell us that the patient is less likely to have knee osteoarthritis and vice versa. In other words, knee osteoarthritis becomes dependent on joint trauma within a sample of patients who undergo surgery (even though they are independent in the wider population).
So, colliders induce bias when they are controlled for, whereas confounders induce bias when they are left uncontrolled. To eliminate both of these biases, we need to identify as many confounders as we can and control for them during the analysis, while at the same time identifying all colliders and leaving them uncontrolled.
Establishing causation from association
Associations can represent causal effects, but only when we adequately control for all confounders, do not control for any colliders, and establish temporal precedence of the exposure and outcome. Even then, unknown confounders and colliders and other biases may vitiate our conclusion. Ruling out confounders and colliders is a key step in establishing causation but is insufficient alone. Use of classical frameworks, such as those of Bradford Hill or the more modern counterfactual framework, will be required to make stronger causal claims.
Without careful consideration of all the above, we’re left with just another distorted association in another dataset.
Hopin Lee, NHMRC postdoctoral research fellow, Nuffield Department of Orthopaedics, Rheumatology and Musculoskeletal Sciences.
Jeffrey Aronson, clinical pharmacologist and Fellow of the Centre for Evidence-Based Medicine; President emeritus of the British Pharmacological Society.
David Nunan, senior research fellow, Centre for Evidence-Based Medicine.
Conflicts of interest:
HL: None declared
JA: None declared
DN: has received funding from the NHS NIHR SPCR programme and the Royal College of General Practitioners.
Disclaimer: The views expressed in this article represent the views of the author and not necessarily those of the host institution, the NHS, the NIHR, or the Department of Health.